A Trade-signal Generator for Improving Portfolio Returns of Factor Strategies
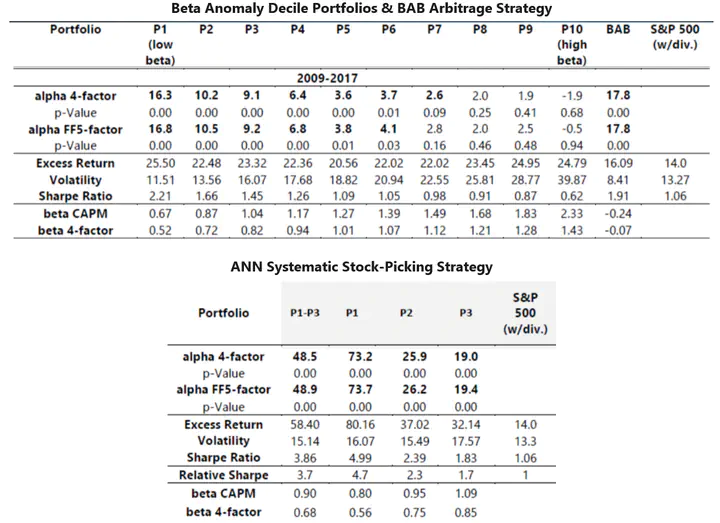
In this project I employed neural networks to explore whether I can increase the realized portfolio return obtained from the long-component of the academically motivated low-beta factor strategy in the US equities space. The network was set to forecast the next-day trade direction signal of several pre-selected stocks –the pool of low-beta stocks. The idea of employing an ANN signal generation model, was to add a second layer of improvement on the already over-performing stocks as implied by the market anomaly and the underlying factor strategy. The network successfully detects buy signals allowing to “hand-pick” several stocks from an already well-performing pool of stocks. My ANN-based investment strategy systematically achieves portfolio gains (as captured by increases in risk-adjusted returns) over the benchmarks. In particularly, over the period 2009-2017, my algorithmic strategy on average increases the Sharpe ratios by 65% and triples the FF5-alphas compared to the standard overperforming low-beta factor decile portfolios (P1-P3). My best performing ANN portfolio (comprised of the 330 lowest-beta US stocks, before “hand-picked” by the network) achieves a 126% increase, resulting a Sharpe ratio of 5, translating to a ratio of 7.7 relative to the Sharpe of the S&P500 with dividends reinvested.